Applications have just opened for the UCSF-UC Berkeley Joint Program in Computational Precision Health’s (CPH) first Ph.D. cohort, which will matriculate in fall 2023. “It’s one of the best computational schools in the world and one of the best medical schools in the world coming together to create this interdisciplinary ecosystem,” said Adam Yala, one of three assistant professors in the inaugural group of core faculty for the CPH program.
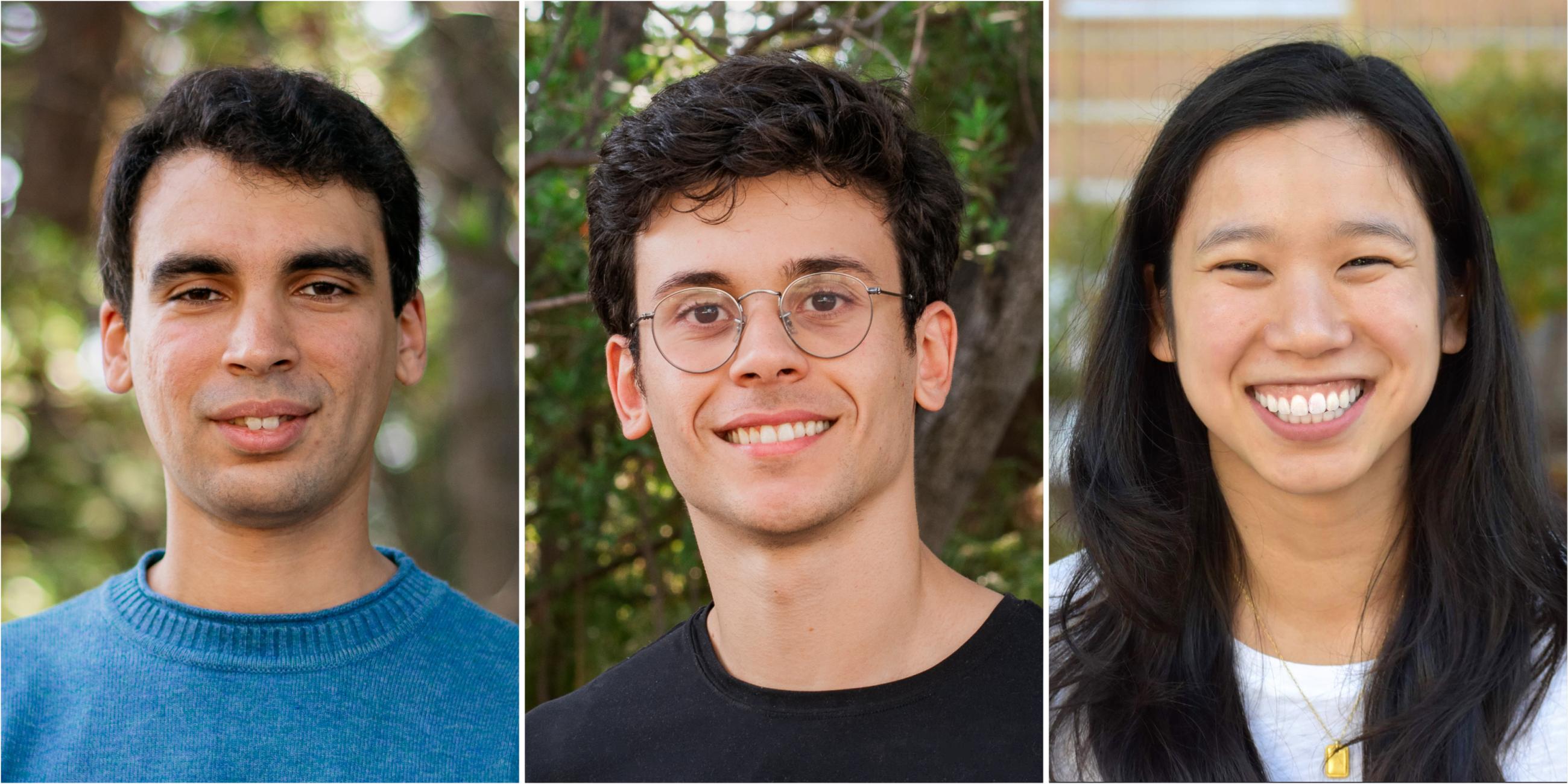
Yala and Ahmed Alaa joined the program this summer, and Irene Chen will join in July 2023 after completing a postdoctoral fellowship with Microsoft Research New England. CPH also draws on the expertise of 39 participating faculty from departments and schools at both universities, including Electrical Engineering and Computer Sciences, Medicine, Epidemiology, Pediatrics, Statistics, and Public Health.
Chen, who described pursuing a Ph.D. as “an exercise in discovery,” said that this diverse and dynamic intellectual environment will help students leverage many different methods and perspectives as they shape their own areas of impact in computation and health.
CPH is distinguished by an intentionally interdisciplinary design, combining computational sciences and health sciences with the goal of creating more robust, personalized and equitable techniques to prevent, diagnose, treat and manage disease. Announced in October 2021, the program is administered in partnership with the Division of Computing, Data Science, and Society at Berkeley and the Bakar Computational Health Sciences Institute at UCSF.
Defining an emerging field with diversity, equity and inclusion at the center
Though not clinicians themselves, both Yala and Alaa were influenced by cardiologists in their families. Yala is from Algeria, where his mother had a cardiology clinic on the ground floor of his childhood home in Algiers. He recalls coming home from school in the afternoon and attempting to interact with patients, often unsuccessfully. “A very loud child is not exactly what you want to be dealing with in your patient waiting room,” laughed Yala.
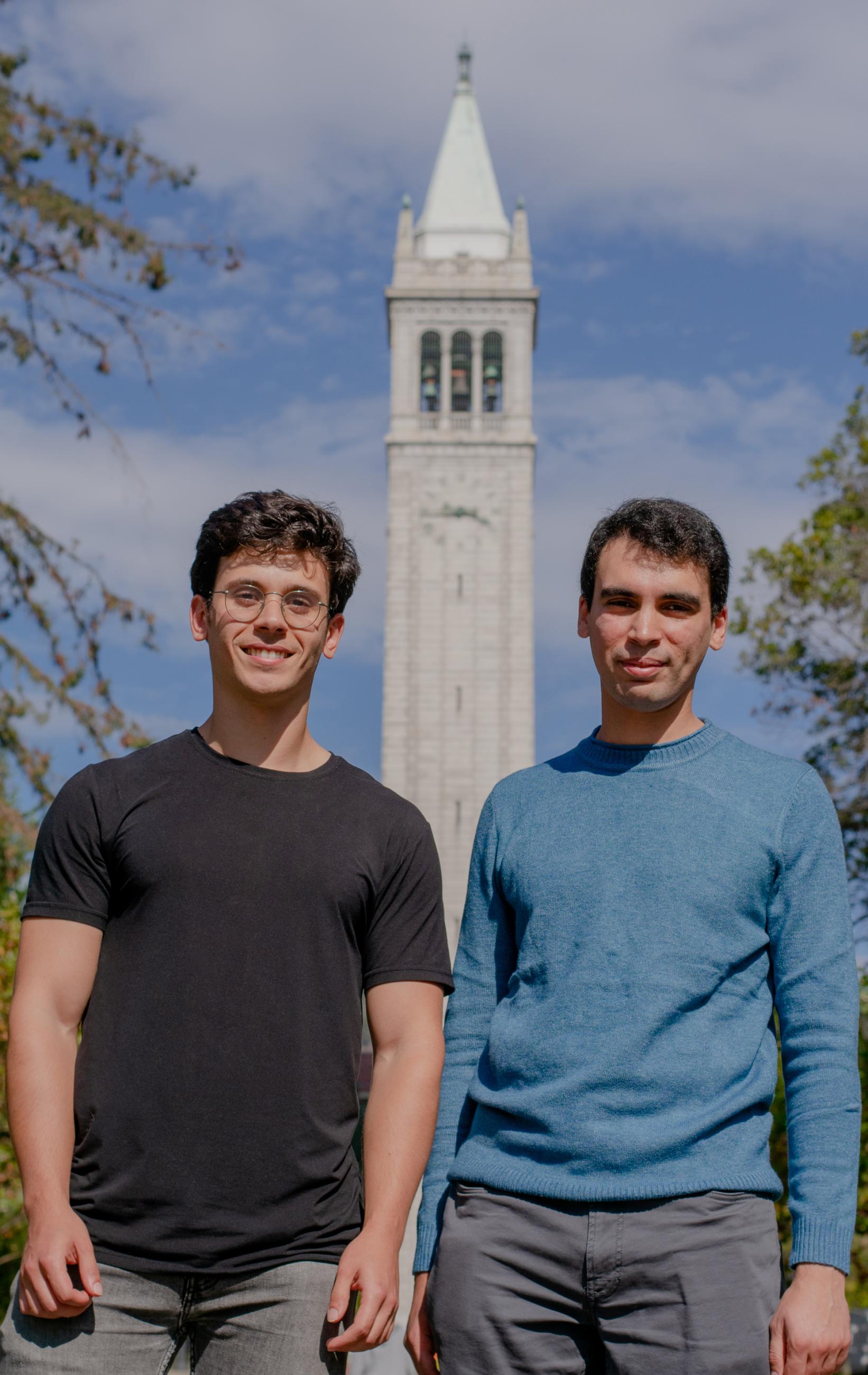
The importance of his mother’s work made a strong impression, but he knew he did not want to become a medical doctor, working with patients on a one-on-one basis. Yala aspired to make an impact on a large scale and become an innovator, though he did not, as a child, imagine this would lead to academic research. In hindsight, the emerging field of computational precision health is exactly what he hoped to find.
The cardiologist in Alaa’s family was his grandmother, whom he described as one of the first women heart surgeons in his native country Egypt. Following in her footsteps, cardiovascular diseases are his primary research interest. The experience of Alaa’s grandmother helped him first grasp the concepts of diversity and equity as he recognized the obstacles she faced in a profession almost entirely dominated by men when she began a career in the 1960s.
Alaa defined computational precision health as “the science of building computational models to capture heterogeneity in clinical care,” where heterogeneity encompasses a broad range of features that vary from patient to patient, including response to therapies, physiological processes associated with disease, differences in care across demographic areas and differences in clinical practice.
Alaa sees diversity and equity as fundamental concerns for this field because data is inherently biased: patients more prevalent in data dilute researchers’ understanding of underrepresented patients. For example, patients without health insurance or those who find the health system challenging to navigate due to language barriers may be less likely to seek medical care and, therefore, less represented in clinical data. Alaa expressed this crucial problem succinctly: "Biases affect data, and precision is learned from data. How can we make sure that the learned precision from data is not transformed into biased models?"
How algorithms may counter or exacerbate existing inequities is a particular focus of Chen’s research. Echoing Alaa’s question, she asked, “What does it mean to deploy a healthcare algorithm and make sure that it is helping people instead of hurting them?” The COVID-19 pandemic emphasized the existence of great needs that the health care system does not currently meet, said Chen. She believes the careful application of computational tools can positively impact the whole patient population.
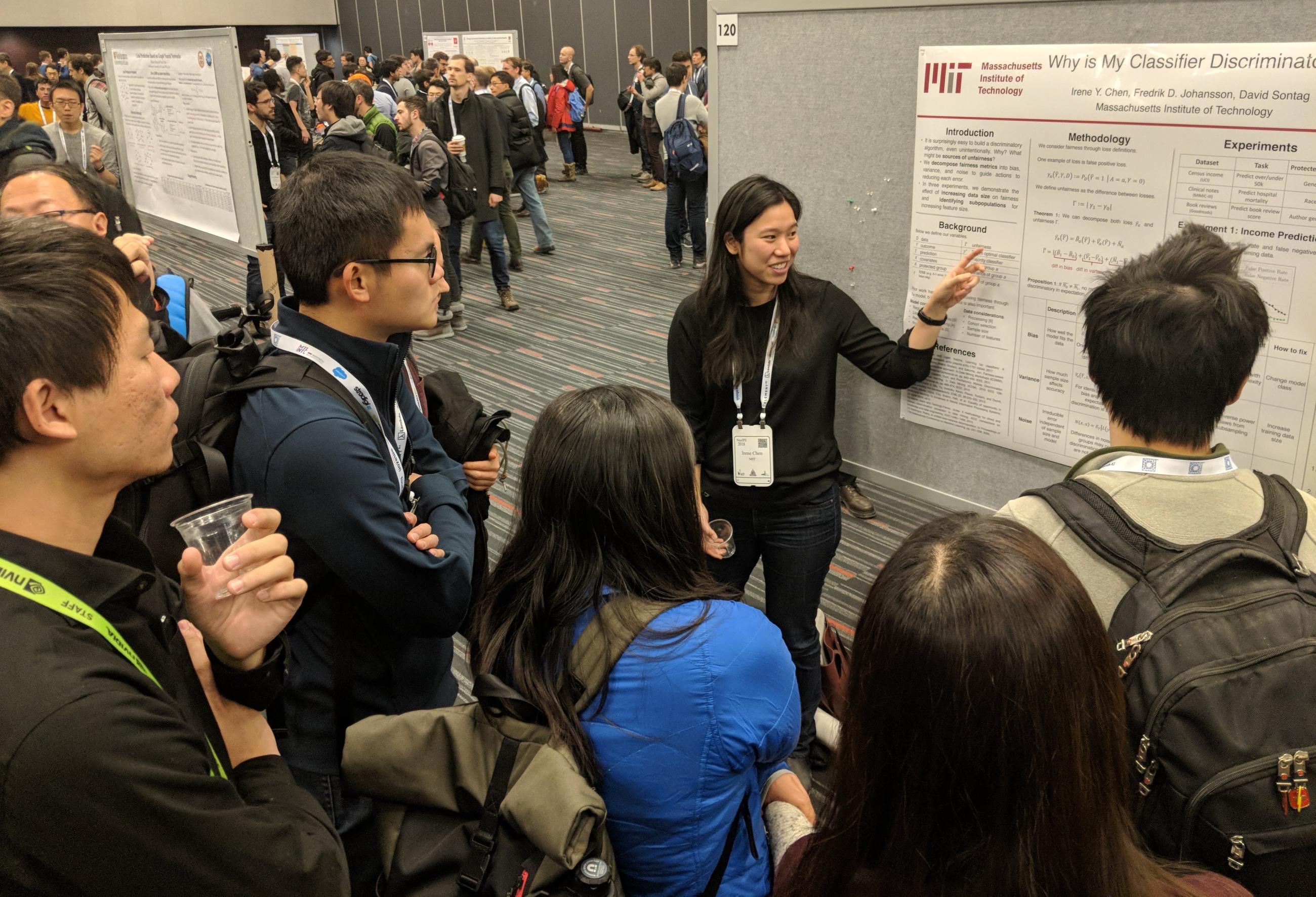
Growing up, Chen was empowered by scientists in her family: both her parents were professors at Georgia Institute of Technology, where her mother was the first woman to receive tenure in the physics department. Chen was named after physicist Irène Joliot-Curie, Marie Curie’s daughter. By the time she was 12, her parents asked her to look over their papers and grant applications, partly because English was not their first language and partly to open a window for their daughter into the world of scientific research.
One of Chen’s most meaningful projects began a few months into the pandemic when shelter-in-place restrictions led to reports of increased intimate partner violence. This type of violence is often understudied because victims may be reluctant to come forward for many reasons, including feelings of shame, social stigma, fear of retaliation or economic dependence. Chen and her collaborators worked with Brigham and Women's Hospital in Boston to identify predictive factors in the medical records of patients known to have experienced intimate partner violence. They developed a machine learning model that assesses radiology reports to predict the likelihood of intimate partner violence and injury and are working with radiologists and emergency room clinicians to refine the algorithm so that clinicians can better support these patients.
Navigating obstacles to research and collaboration
Alaa, Chen and Yala agree that data could be better leveraged to improve care for patients, both now and in the future. Only a small percentage of patient data informs patient care, and the record of one patient’s experience doesn’t necessarily lead to a better experience for the next patient.
Despite the volume of data available, researchers face significant challenges, from gaining access to electronic health records to implementing tools in clinical settings and the siloed nature of much scientific research. As a computer science Ph.D. student, Yala individually reached out to more than 50 hospitals to gain access to data, even recruiting friends to send inquiry emails on his behalf. There was no established pathway connecting him to hospitals or clinicians, leading him to devote a great deal of time, as well as “pure willpower and hustle,” to push forward a project that uses mammogram images to predict the risk of breast cancer. The resulting risk assessment model showed consistent performance across datasets from the U.S., South America, Europe and Asia.
"In many cases, we could have significantly reduced patient suffering if only we caught the disease earlier," said Yala. "By building better technologies, we have the opportunity to systemically change outcomes."
Like Yala, Alaa said the most time-consuming aspect of his research has been seeking collaborators and patient data. Alaa’s previous projects, which include a clinician-facing breast cancer prognosis application and a COVID-19 hospital capacity forecasting system developed with the National Health Service in the U.K., drove home the complications of developing and implementing computational tools aimed at achieving a real-world impact. The cost of that work is much higher if you’re not in an ecosystem already geared toward interdisciplinary research, said Alaa.
Creating an interdisciplinary ecosystem with expansive resources
Alaa has already had a distinctly different experience with interdisciplinary research at CPH. Within his first month, he began a collaboration with Berkeley biostatistics professors Mark van der Laan and Maya Petersen, who co-directs the CPH program with Ida Sim of UCSF. Supported by a philanthropic donation from the pharmaceutical company Novo Nordisk, they are collaborating with researchers from the University of Copenhagen, Oxford and other institutions to develop methods for combining clinical trial data and hospital observation data to improve sample size and correct for potential sources of noise and bias. If successful, this work could aid research on many different diseases.

“One of the misconceptions about research is that you do it in a vacuum and that there’s this lone genius who churns out papers and ideas,” said Chen. “In fact, so much of the work in this field is interdisciplinary and collaborative. It has to be by the very nature of what we study.”
Chen was drawn to CPH partly because of the unparalleled opportunity to access deidentified electronic health records from over seven million patients from all five UC medical centers. And since CPH is embedded in UCSF’s world-class clinical environment, the program offers researchers firsthand insights into clinician-patient interactions and pathways for testing and deploying AI and machine learning tools in the real world. “We have a unique opportunity to actually ground all our new algorithms to meaningful practice,” said Yala.
Alaa said the program is uniquely positioned to create a much-needed bridge between disciplines, helping students with medical backgrounds understand the computational world and how it interfaces with clinical settings and providing computation students with the opportunity to understand and confront real-world clinical problems. He added that these diverse and complementary skills are in high demand and hard to achieve in mainstream programs.
Yala added, “I truly believe that through methodological innovation we can make better care, and I think it's a uniquely well-positioned program to have that kind of impact.”
The UCSF-UC Berkeley Joint Program in Computational Precision Health is open for Ph.D. applications until January 6, 2023. For more information, visit the CPH program website.