Microsoft’s prime unifying scientist has a message: “Artificial intelligence” does not exist.
At a Sept. 13 lecture at UC Berkeley, Jaron Lanier urged the audience to look past what they learned from science fiction as children and stop treating AI systems as entities. Instead, they should talk about the tools as social collaborations trained on materials by people.
“It doesn’t matter technically,” Lanier said. But “if you analyze it on those terms, you have a much more actionable way of understanding it, and if you integrate it into society on those terms you have a brighter and more actionable set of paths open for the future of society.”
Artificial intelligence has become a hot-button issue following last year’s release of a new version of ChatGPT, a chatbot underpinned by a large language model (LLM). Lanier argued that by re-framing the debate, we could open the door to solutions for issues like job displacement and create a more inclusive vision of the future.
The lecture was the first of four events in a fall speaker series sponsored by the Center for Information Technology Research in the Interest of Society and the Banatao Institute, Berkeley’s College of Computing, Data Science, and Society and the Berkeley Artificial Intelligence Research Lab. The series explores discoveries, societal impacts and the future of AI and highlights Berkeley’s leading role in this space.
‘Hey, bot. Why so weird?’
Technologists could support a re-framing on AI by changing how these systems are trained, Lanier said.
One clear rule that a model can follow to make apparent the human-centered, collaborative nature of these tools? Teach a bot to share the most essential sources that it used to construct its response. So when a chatbot does something strange like declare love for a journalist, people could ask what information that note is based on and alter the inputs.
“You can say, ‘Hey, bot. Why so weird?’ and it would say, ‘Oh, this is fan fiction and soap operas, don’t you like it,’ and you can say, ‘No, leave that stuff out. This is supposed to be a fake essay for my college class,’” said Lanier. “I’m not suggesting it’s the universal solution or the only solution, but it’s the only concretely defined solution.”
Highlighting the importance of humans as sources for, and the fuel of, this kind of technology could alleviate some public anxieties around role displacement, Lanier said. It could also offer opportunities to improve the tools.
Chatbots are already passing high-level medical, legal and business exams, producing art and writing news articles. This has spurred fears and aspirations that paid work may go away and be replaced instead by a universal basic income, Lanier said.
This is a political problem in that the institution distributing that income would consolidate immense power, the kind of power that “the worst people” typically try to seize, Lanier said. It’s also a spiritual issue, leaving some individuals feeling disenfranchised and devalued, he said.
“We’re [the tech industry] creating a future that repels most people, a future that makes them feel unloved, unneeded and left behind,” said Lanier. “If we call it a social collaboration rather than a new creature on the scene, that problem goes away.”
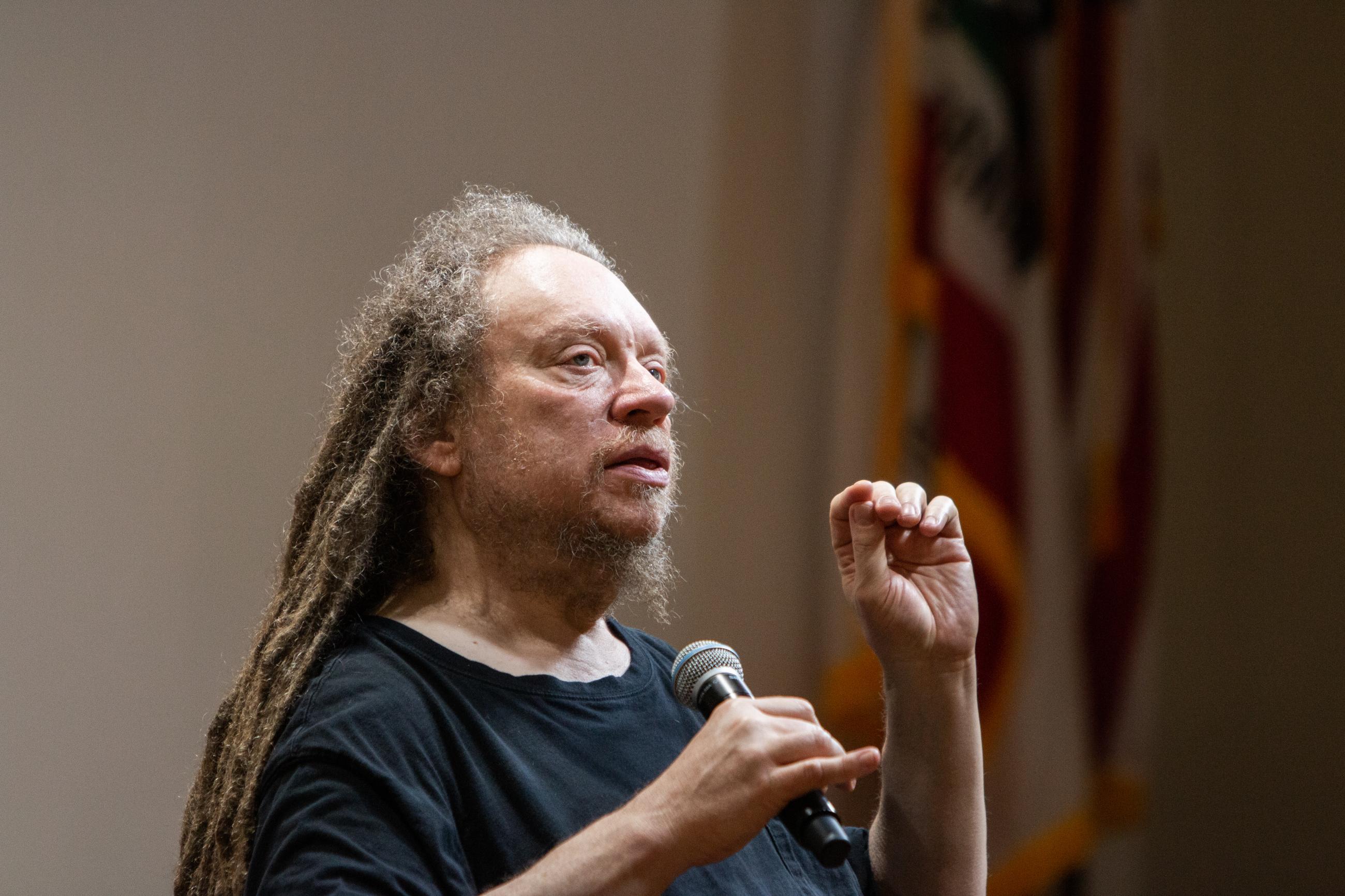
A new creative class
Instead, Lanier urged the audience to champion humans’ value to improve these models. People might even be paid by one another in a new kind of marketplace to create hard-to-find information that would fill in gaps in models’ training data, he said.
This would provide work for some people who experience a loss due to AI and improve these technologies, Lanier said. It would also encourage broader participation in the data economy and put “more value on the books,” which benefits everyone, he said.
“Why not create a new creative class instead of a dependent class,” he asked. “There’s a general direction in data dignity that offers us a human-centered future that just has more concrete terms for improving the technology and more positive terms for the human roles in it, and the whole thing makes more sense.”
These approaches are not without challenges. Changing how chatbots are trained when the tools are already “crazy successful” is a hard ask, for example, Lanier said, and getting people to approach AI as social collaborations instead of entities is an uphill battle.
Tackling the challenges could make these tools more useful and equitable. Take the training data that LLM tools learn from, which is largely from white, western individuals, an audience member said. How could that be expected to work equitably for everyone? they asked.
Lanier responded that the antecedent data should “reflect humanity,” and it’s possible to do that. One key tool is to look at where the data is coming from – who the collaborators are – not just what responses come out of these kinds of systems, he said.
“Why can't people be motivated to make the training data work better for society?” Lanier asked. “To me, this would be a sterling example of where it should.”