Researchers from UC Berkeley and UC San Francisco pursuing new approaches to computational health convened an April 29 conference to share progress and challenges in connecting disparate healthcare data to improve clinical decision-making and patient outcomes.
“We all understand that technical solutions to problems are not going to be any good if we don't have ways of scaling them up, ways of delivering them that are ethical and accessible to the broad public,” said Kathy Yelick, vice chancellor for research at Berkeley, in opening remarks.
The Frontiers of Computational Health conference gathered more than 150 academic and industry researchers, staff and students from disciplines across varied health specialties, computer science, engineering, and social and behavioral sciences. The interdisciplinary group discussed the latest computational approaches and methods for disease detection, risk prediction, decision-making and more.
Held on the Berkeley campus, the conference included panel discussions and poster sessions organized by the UCSF UC Berkeley Joint Program in Computational Precision Health (CPH), the UCSF Bakar Computational Health Sciences Institute, Berkeley’s Center for Targeted Machine Learning and Causal Inference, and Berkeley’s Center for Healthcare Marketplace Innovation.
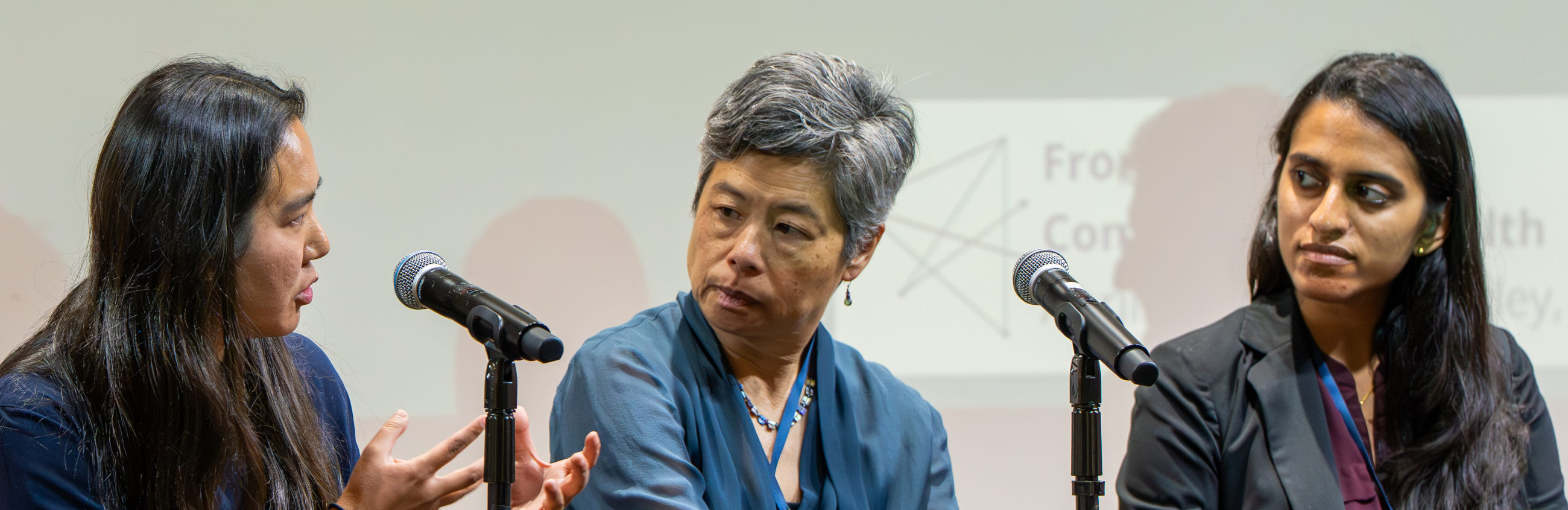
Using AI in research while protecting scientific integrity
Eric Horvitz, chief scientific officer at Microsoft, gave a keynote talk on the cumulative history of research in machine learning that has enabled recent advances in healthcare applications, including clinical decision support that combines input from multiple data sources and modalities. While considering the widespread proliferation and use of generative AI models in the last few years, he referenced a Proceedings of the National Academies of Sciences editorial he co-authored with recommendations for using AI in research while protecting scientific integrity.
“We’re going to rely on a constellation of analytical methods where we continue to leverage and move forward applications with classical frameworks, while keeping an eye on advances with neural network models and also seek new syntheses that I expect with the maturation of AI,” Horvitz said.
Modeling physician decision-making
Jon Kolstad, an economist at Berkeley and faculty director of the Center for Marketplace Innovation, presented recent work on physicians’ clinical behavior and decision-making. The research team includes biostatistician and CPH co-director Maya Petersen, PhD student Jonas Knecht, and researcher Anna Zink.
The team is building a unique foundation model trained on physician action logs, capturing the dynamic and complex process of treatment decisions. Kolstad discussed this new approach to understand and interpret a doctor’s implicit knowledge gained from experiences working in an emergency room, for example, that they use to make multiple decisions in caring for each patient.
“Can we measure how thinking changes within a specific doctor as a function of their cognitive resources available?” Kolstad asked. He said the team discovered that a high cognitive load for the doctor increases the number of potentially unnecessary diagnostic tests for the patient. This new foundation model could lead to a better understanding of physician decision-making in different situations, and ultimately to interventions that improve patient testing and outcomes.
“Our approach is basically to build a model that captures the dynamic and complex process of treatment decisions,” he said. “We're thinking that learning this [decision-making] path actually embeds an incredibly rich amount of knowledge that a physician has.” With the ability to predict clinical actions, the model could capture what Kolstad called “fundamentally the language of medicine.”
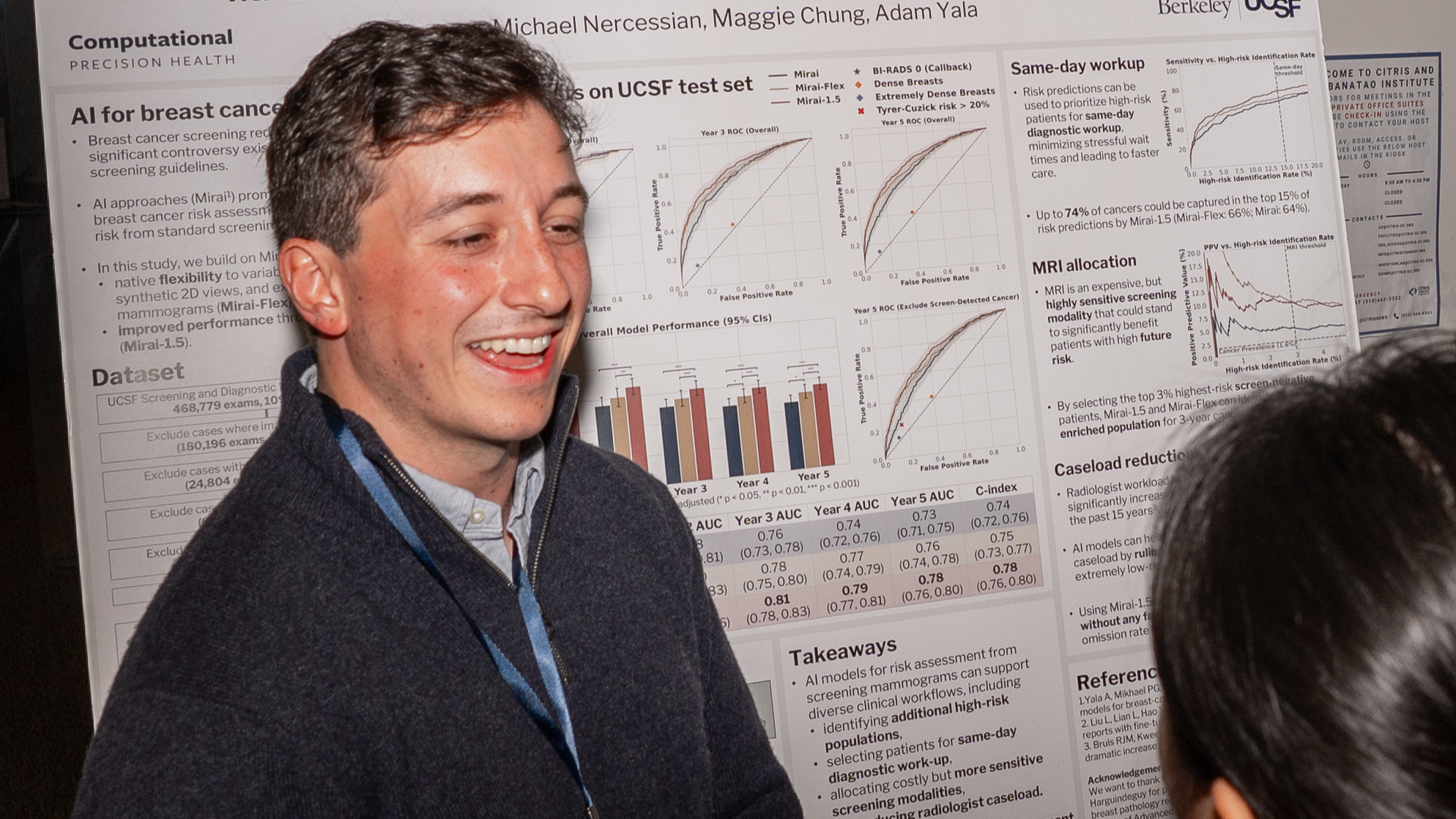
Managing health data for accessibility and security
CPH co-director Ida Sim spoke about the opportunity to drive AI-driven discovery by integrating real-time data in ways that personalize patient care and make earlier health interventions possible. As a primary physician and informatics researcher, Sim expressed a strong desire to help her own patients and others maneuver healthcare systems that are incredibly siloed.
Sim is working with Berkeley statistician Fernando Pérez to build JupyterHealth, an open software platform and secure environment for collecting, managing and analyzing health data that’s accessible to researchers, clinicians and patients.
“One of the insights I got from my early work is that patients need to get value from the data and the effort they put in,” said Sim. “We need to think about: what are the insights they want that answer their questions, and then how do we get this information back to them.”
Collecting patient data with wearable sensors
Sandya Subramanian shares Sim’s interest in connecting with patients. An assistant professor in computational precision heath at Berkeley and UCSF, Subramanian’s translational research includes developing wearable sensor systems to collect clinical-grade data in non-clinical settings, enhancing machine learning and AI models to interpret physiological signals, and creating AI tools informed by physiology.
Subramanian discussed a study she led monitoring patients’ unconscious perception of pain during surgery while under anesthesia as an example of sensor-based systems that can detect physiological signals of which the patient is unaware. She’s also working on non-invasive wearable sensors to monitor sleep-wake behavior and other indicators that could be used to track disease and health recovery in a patient’s own home.
“I think the holy grail in every situation is to work toward personalized prediction,” Subramanian said.