Building Machine Learning Models for Arctic Ice Restoration
The recent decades decline of the multi-year sea ice pack show that the emerging Arctic
may be beyond the point of recovery by only reducing the GHG emissions and urges for
additional mitigation measures. Arctic Ice Project (AIP) has proposed a technology that
increases the brightness of the sea ice enhancing the sea ice albedo and thus reducing the
sea ice melt. Climate modeling by Climformatics, Inc. has shown promising results for this
technology to slow down the accelerated rates of Arctic ice melt due to Global warming. The
primary goal of this project is to develop ML models of the relationship of the Arctic surface
albedo and the sea ice pack characteristics such as sea ice thickness and area, using
results from climate model simulations and observations. We will also investigate the
sensitivity of these models to the Arctic cloud cover at different vertical levels (low, mid- and
high-clouds). This will help to determine where and when AIP surface albedo enhancement
will have the largest impact for the Arctic ice pack restoration. The students will use
advanced methods of machine learning and data science to analyze climate model output
data sets and observational data sets (satellite or in-situ) for albedo and cloud fraction.
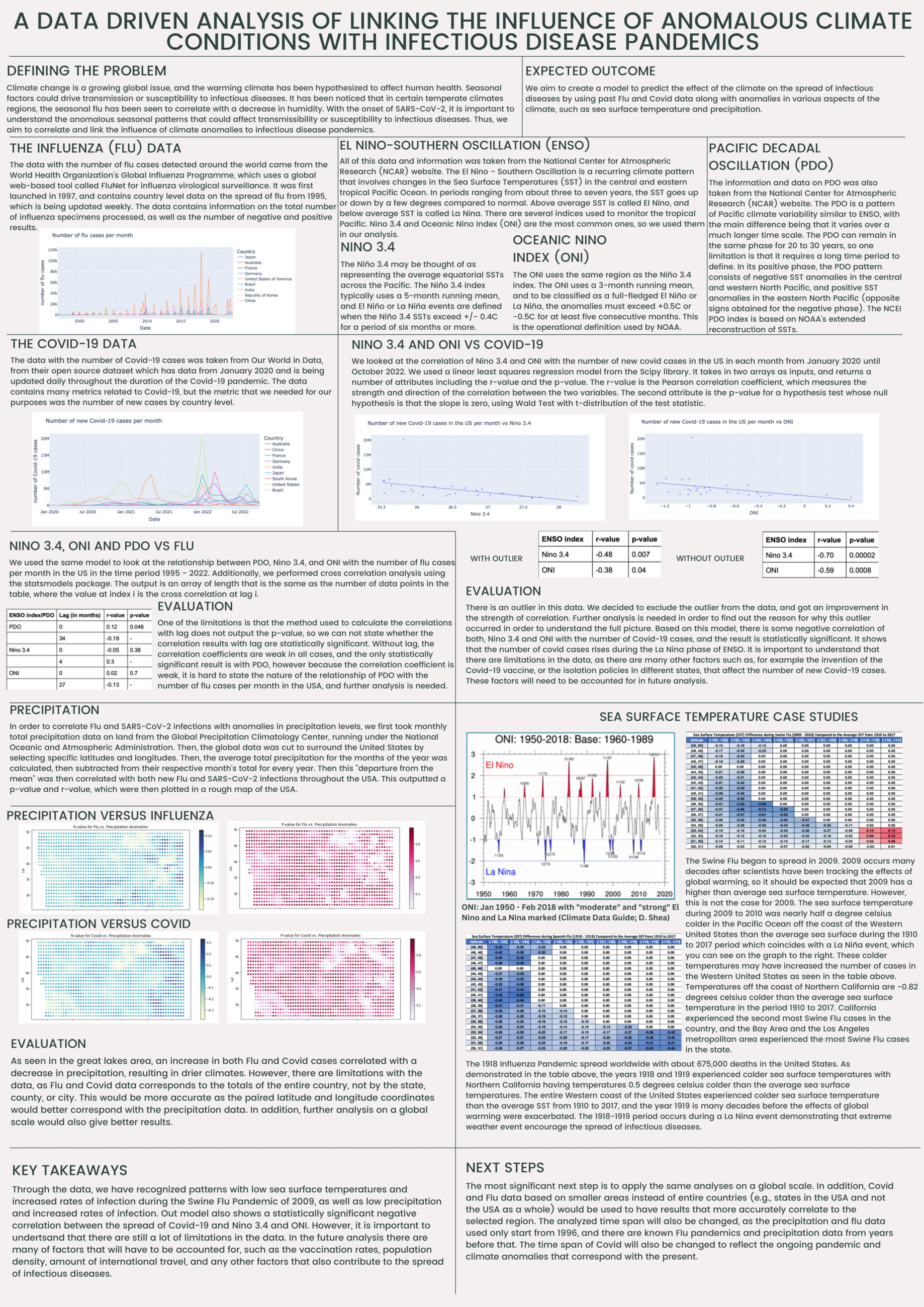