The mission of the Bureau of Ocean Energy Management (BOEM) within the Department of the Interior (DOI) is to manage development of U.S. Outer Continental Shelf (OCS) energy and mineral resources in an environmentally and economically responsible way. The Pacific Region manages these resources in federal waters off the coasts of California, Oregon, Washington, and Hawaii. In the Office of Strategic Resources, part of our responsibility is to manage existing oil and gas leases, and appropriate access to oil and gas resources through resource evaluation, reserves estimation, and geological and geophysical data acquisition. In addition, in 2021 the Infrastructure Investment and Jobs Act was signed into law which gave the Secretary of the Interior the authority to grant a lease on the OCS for long-term carbon sequestration. Our section leverages geological and geophysical data (often collected 20+ years ago) to satisfy BOEM mission for the Pacific Region.
A 2D seismic line is a geophysical dataset that gives the interpreter an idea of the geology of the subsurface. It is like looking at a picture of a vertical slice of the rocks below your feet. We propose to develop an unsupervised algorithm to separate a 2D seismic line into meaningful sub-regions (seismic facies) based on quantitative waveform characteristics. There are two ways that this can be done: evaluating patterns in the wiggle trace (can extract x,y,z data points that have information in them), or performing the unsupervised learning on a picture of the data. These results would undergird subsequent geological interpretations for a variety of applications.
Quantitative seismic analysis, which includes seismic facies classification, is not new but ML and widely available computing power may hold much promise. The concept of seismic facies became widely adopted after Exxon geoscientists popularized sequence stratigraphy in the 1970s. See this webpage for more information on seismic facies (https://wiki.seg.org/wiki/Seismic_Facies_Classification). By the early 2000s, neural networks were being used to infer sub-seismic scale reservoir properties.
Development of this ML tool is timely and has practical importance. Interest in the Pacific OCS is increasingly the focus of a variety of activities that require understanding of the subsurface geology. Examples range from carbon sequestration feasibility studies to geohazards assessments at floating offshore wind farm sites. Robust, quantitative determinations of seismic facies using ML techniques would support geologic interpretations for these types of OCS activities.
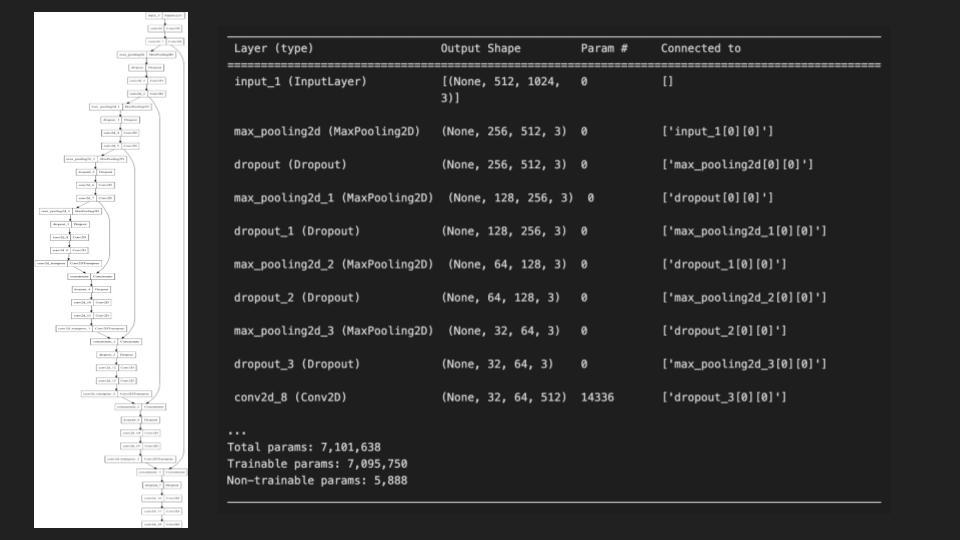