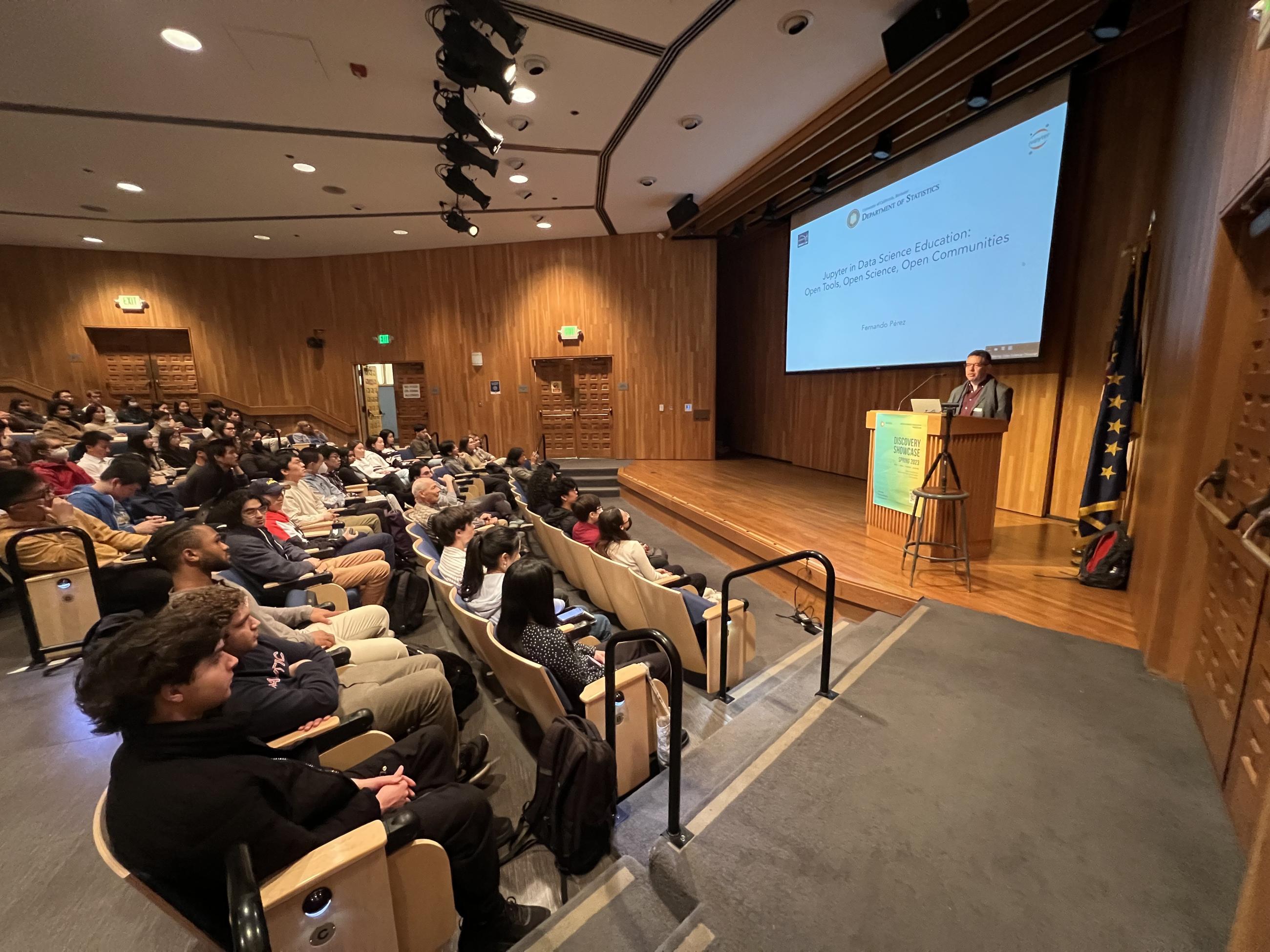
The Spring 2023 cohort of Discovery is the largest cohort ever with more than 110 projects and 400 students joining the Data Science Discovery Program this semester. On Wednesday, May 3rd at the Sibley Auditorium in Bechtel Engineering Center, students displayed the work they did throughout the semester and the results of their research. Students, faculty, staff, and external visitors all came to gather the insights from their work and listen to a keynote speech by Professor Fernando Perez.
The recording of the speech and presentations can be found here.
Of the 110+ teams that participated this semester, five teams were recognized with awards while five others were recognized as runner-ups. The five categories for this semester are the Data Science Insights Award, Ribbon of Excellence, Cloud Computing Application Award, Data Visualization Award, and Team Collaboration Award.
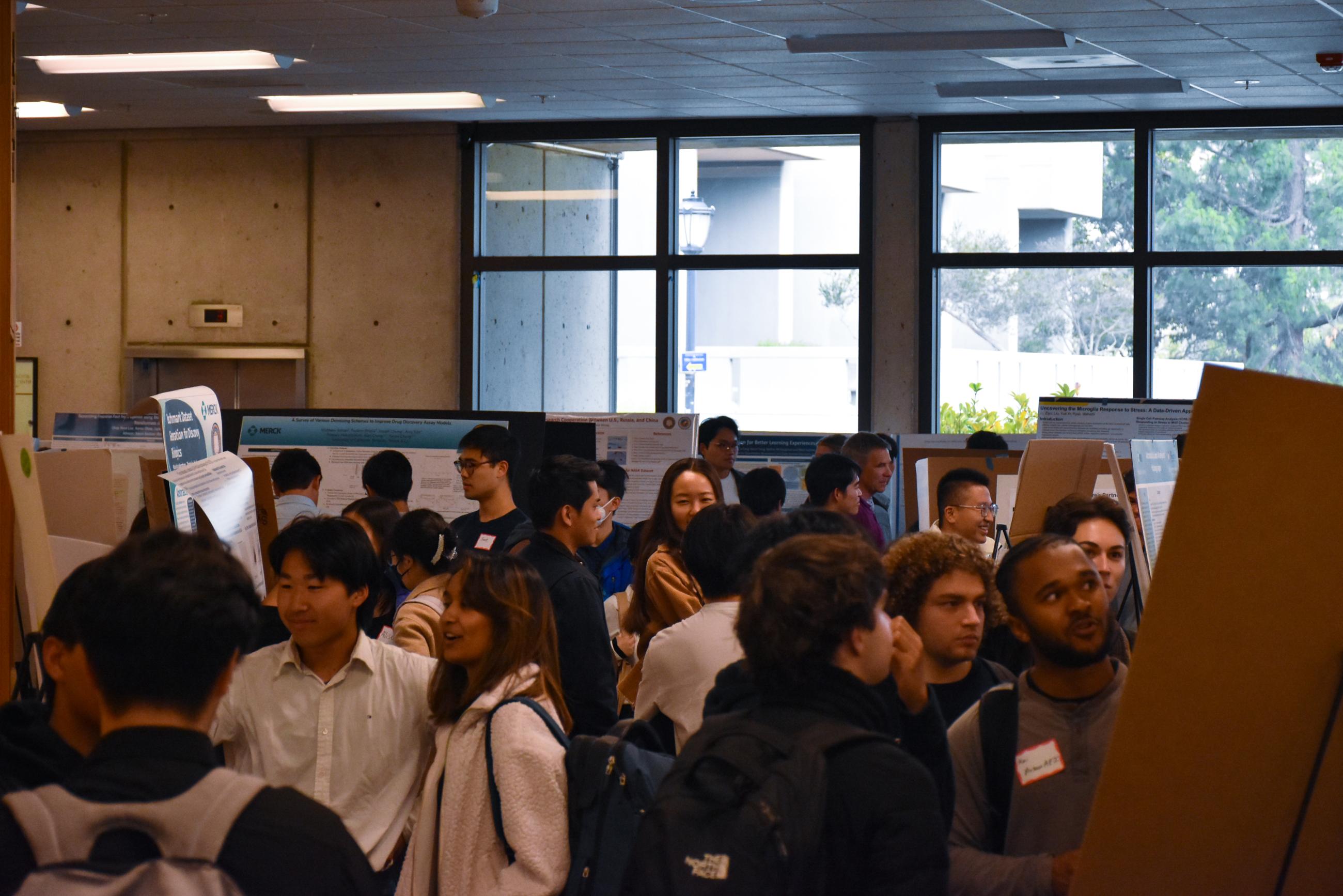
The Data Science Insights Award went to the “Building Allele Analyzer from Human Genomes for CRISPR Gene Therapy” team. Student Patrick Issagholian-Lewin worked with Claire Clelland’s team from the Clelland Lab at the Weill Neurosciences Institute in the Department of Neurology at UCSF to create “a toolkit that allows researchers to account for the uniqueness of individual genomes when developing therapies,” according to Patrick’s poster. The project focuses on finding CRISPR guide RNAs and gRNA pairs that can provide the most widespread coverage of a sample or population, which can be incredibly useful for researchers building therapies for diseases that were once thought to be incurable.
The “Building Allele Analyzer from Human Genomes for CRISPR Gene Therapy” received the Data Insights Award due to its unique and compelling insights and clearly outlined methods. The team detailed the steps taken using a flowchart and highlighted the most frequent RNA guide pairs that cover 50% of sample genomes. The final pipeline creates a ranking of guide pairs based on sample coverage, allowing for more effective therapies. The team also outlined a system to allow for future progress and local access, giving their insight potential to be a significant breakthrough in the intersection of medicine and data science.
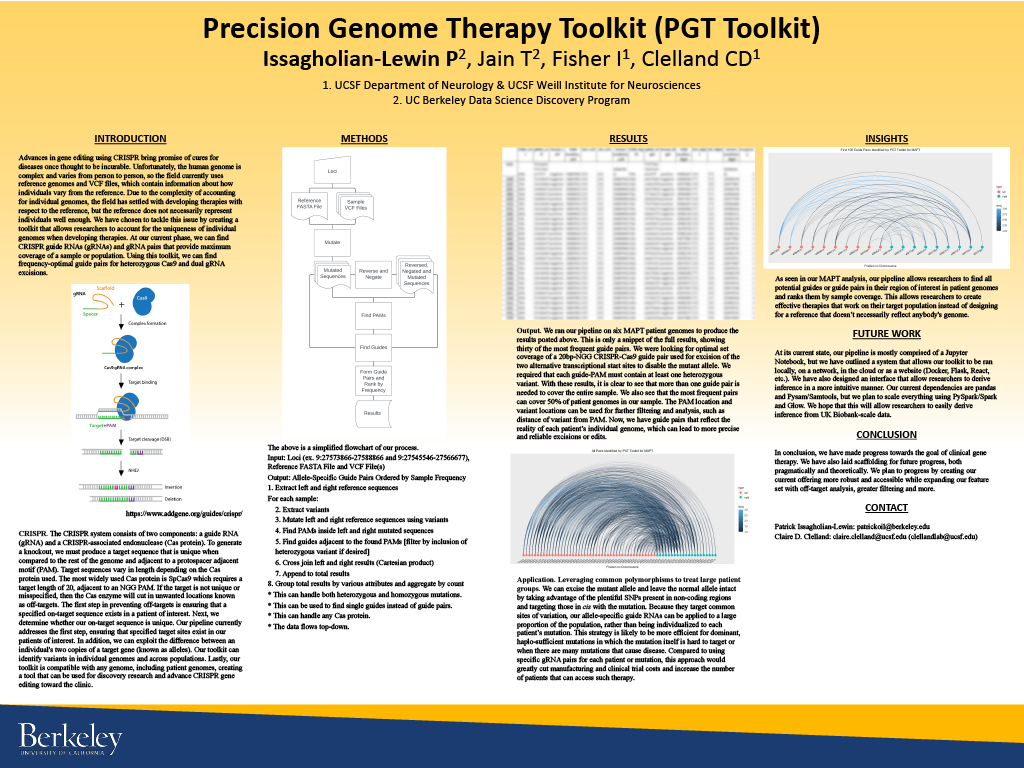
The runner-up for the Data Science Insights Award was the “Exploring Animal Behavior Classification Methods” team. Students Zhiqin Chen, Alex Cao, Enran Wu, and Sai Kolasani worked with Changwan Chen’s team from UC Berkeley’s Dan Lab to assess how animal behavior changes with neurodegeneration and circuit dysfunction. The team highlighted their method for automatic animal behavior classification and concluded that their final classification method provides a more efficient and consistent behavior assessment method.
The Ribbon of Excellence went to the “Separating Financial Fact from Opinion Using Bidirectional Encoder Representations from Transformers (BERT)” team. Students Aaron Chow, Chae Yeon Lee, Tom Lee, and Ye Joon Han worked with Adam Badawi from UC Berkeley Law to train a machine learning model to identify and separate facts from opinions in filings maintained by the Securities and Exchange Commission (SEC). Another objective was to analyze earning calls to identify language patterns associated with stock price movements, and to understand how changes in legal consequences related to factual speech have changed the language firms and companies use over time.
This team received the Ribbon of Excellence due to their excellent display of all components of the data science life cycle. The data science life cycle involves asking a question, obtaining data, understanding the data, and using it to gain insights about the project domain to eventually implement solutions based on the insights. The team clearly outlines the objective and details the materials and methods used, including the source of their data and an explanation of FinBERT, the pre-trained natural language processing model that served as the foundation of their final model. Their results show a deep understanding of their data, emphasized by data visualizations and explanations for each one. They produced a successful model with an accuracy level of 0.93 and concluded that the management discussion and analysis (MD&A) sections of financial market texts contained significantly more opinions than the risk factor section. The project highlights how machine learning algorithms and data science can provide significant analysis in the finance sector.
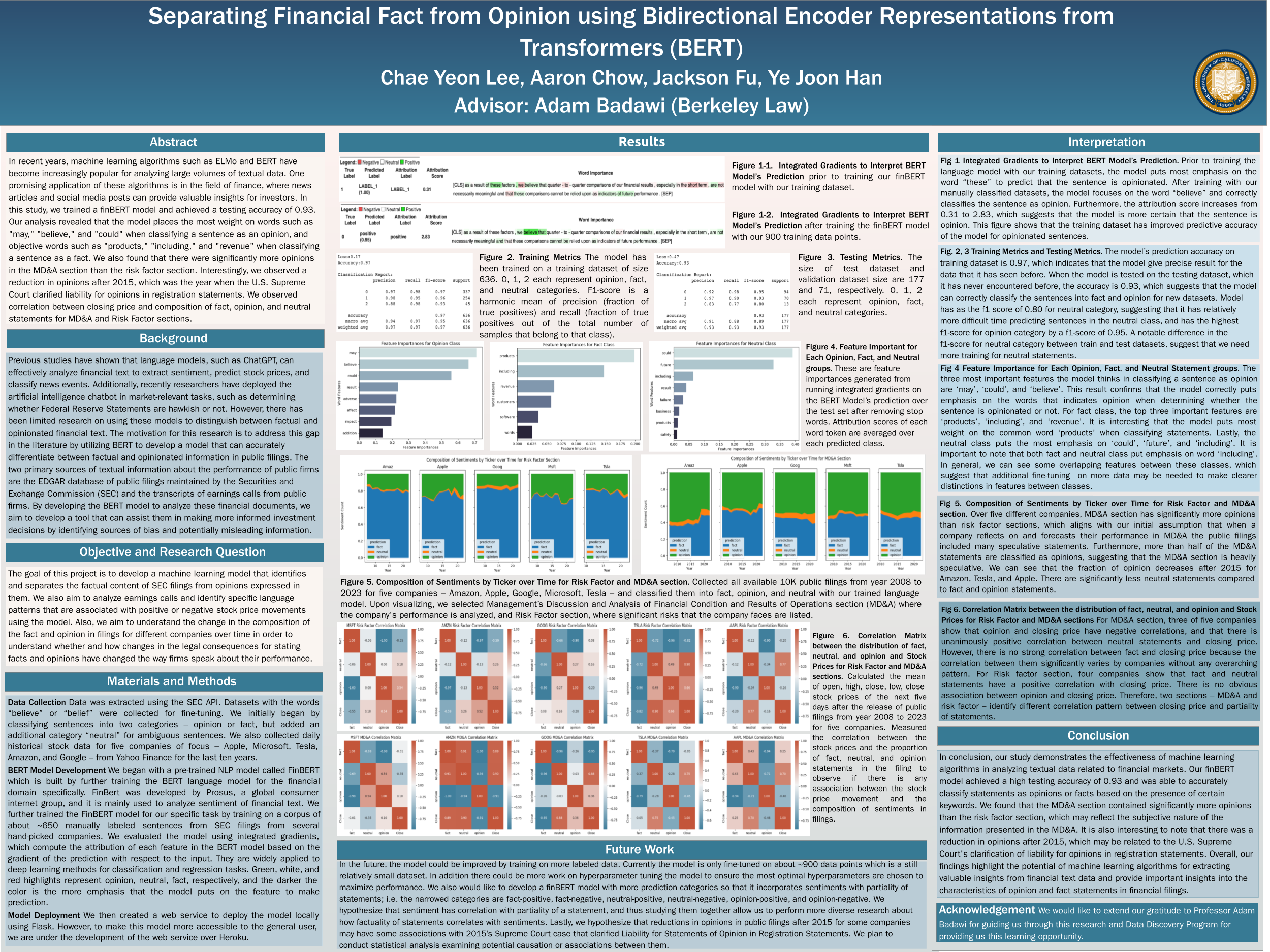
The runner-up for the Ribbon of Excellence was the “Ensuring the Longevity of Just as Special’s Foster Care Resource Database”. Students Brie Zhou, Deheng Peng, Fanyi Lyu, Irene Widiaman, Madeeha Khan, WingYeung Ma, Sophia Zheng, Abbie Tsai, Cindy Zhang, Evie Currington, Katelyn Jo, Richard Zhuang, and Ryan Chen worked with Emmy Tither’s team from Just As Special, a social enterprise dedicated to supporting foster families and volunteers. The team approached the many issues foster families and foster care facilities face in three ways: textual, auditory, and storytelling. The team was able to extract resources and analyze existing data in relevant ways to best utilize the currently existing resource database.
The Cloud Computing Application Award went to the “Faculty Hiring Analysis” team. Student Jeffrey Zhou worked with Karin Garrett’s team at the Moore Accuracy Lab at Berkeley Haas to find the predictability of job performance using scholarly citation counts. The team utilized the OpenAlex database containing millions of authors and their recorded works, selecting a random sample of over 500,000 scholars whose first citation was in 2012 or later. They were able to find a strong predictive validity in the correlation between an author’s performance early in their career and their performance later in their career, but determined that limiting citation counts to a three year period post publication resulted in almost no correlation.
This team received the Cloud Computing Application Award for their relevant usage of Savio, Berkeley’s High Performance Computing Linux cluster. They also utilized Google’s BigQuery cloud resources to analyze over 80 billion data points or over 1.6 TB of data. The team was able to use BigQuery to execute optimized SQL queries and instructions, resulting in a computational time reduction of over 8500 times. By using cloud computing resources to perform tasks and analyses that would be otherwise impossible, the team demonstrated an innovative use of cloud computing platforms.
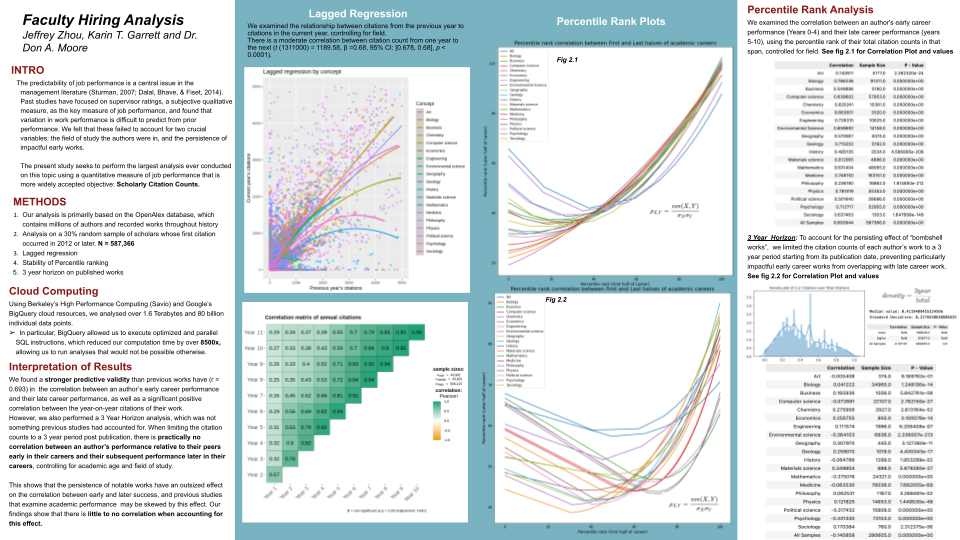
The runner-up for the Cloud Computing Application Award was the “Machine Learning-based Analog / Mixed-Signal Circuit Design and Modeling” team. Students Yuhan Chen, Akira Chou, King Han, Henry Tsai, Aakarsh Vermani, Wayne Wang, and Yifan Zhang worked under the guidance of Vladimir Stojanovic’s team at Berkeley Wireless Research Center to build a cloud-based service for external researchers to access tools for circuit design and test machine learning models for analog/mixed-signal circuit designs. The team used Google Cloud Platform to host their services, mainly for authenticating users and running simulations that would be difficult on consumer PCs.
The Data Visualization Award went to the “High Spatial Resolution Mapping of Emissions and Air” team. Student Niloo Ebrahimi worked with Dr. Ronald Cohen from UC Berkeley’s Department of Chemistry to analyze carbon dioxide emissions in the Bay Area to track aviation emissions and their impact on the carbon footprint of the area. The research focused on aviation datasets from San Francisco Airport, San Jose Airport, and Oakland Airport as well as data about engine types, landings, and aircraft types from the International Civil Aviation Organization. The team concluded that aviation accounts for approximately 2.5% of all carbon emissions in the Bay Area, producing an average of 649 tons of carbon per hour.
This team received the Data Visualization Award for their clear representations of landing data from each of the three major airports in the Bay Area prior to COVID. Additionally, the visualization depicting the sinusoidal approach for future predictions of kilograms of fuel burned per year is clear and shows a clear and easily understood cyclical relationship. The map-based visualization of mean CO2 emissions is also useful for understanding the amount of carbon emissions from aviation activity. The visualizations chosen are meaningful and filled with significant information about carbon dioxide emissions, which is an increasingly important topic related to understanding and slowing climate change.
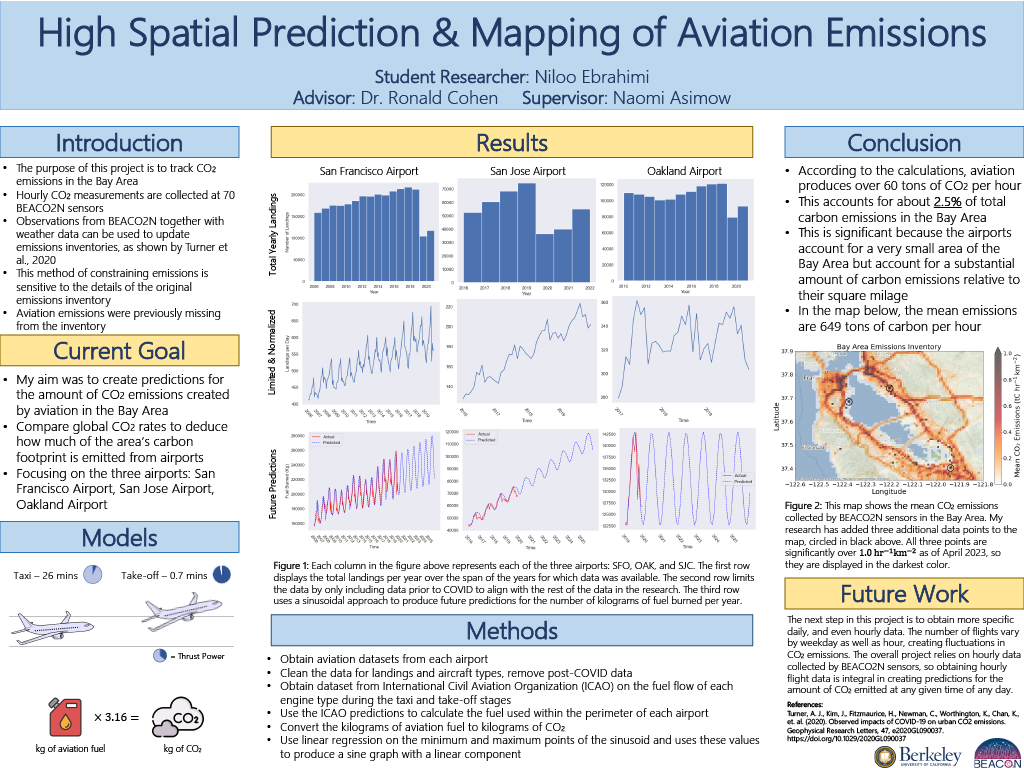
The runner-up for the Data Visualization Award was the “Analyzing Changes in the Affordable Connectivity Program” team. Student Hrag Kouyoumjian worked with Kat Aquino’s team from EducationSuperHighway, a nonprofit organization that provides advocacy and consultation services for school districts to close the digital divide and provide high-speed internet connections in public school classrooms. The team extensively used maps to show the drastic decrease in subscribers from the American Connectivity Program, which provides $14.2 billion in broadband funding for low-income households.
The Team Collaboration Award winner was the “FactGrid Cuneiform Project” team. Students Aaron Ha, Aidan Praytor, Athena He, Brian Hsiao, Canrong Qiu, Daisy Wang, Derry Xu, Floyd Fang, Grace Qian, Haeun Baek, Jack Kwon, Jay Jaisankar, Joreen Li, Joshua Chuang, Julia Wang, Kevin Gao, Lawrence Chen, Lirui Huang, Lydia Wang, Melinee Her, Micaela Montes, Minoo Kim, Mucizhen Zhu, Natalie Wei, Rohit Jha, Saiyra Qureshi, Sanik Malepati, Sriya Kantipudi, Sydney Tung, Tejasv Bhatia, Tina Chen, Tyler Lam, Vinay Vinod, Win Moe, Yuanjue Zhu, Yunze Du, and Zaid Maayah all worked with Adam Anderson from FactGrid Cuneiform to create a JupyterBook to process all Oracc projects and provide further analysis using graph theory and linked data. This team received the Team Collaboration Award for successfully completing research with such a large team and for efficient cooperation efforts.
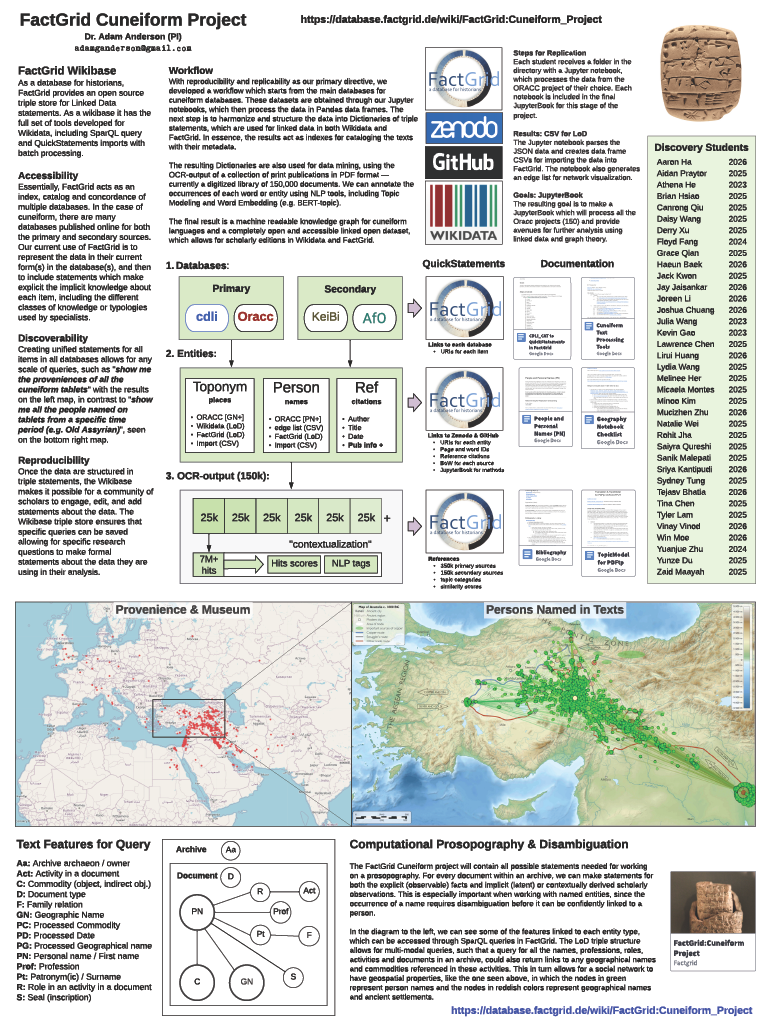
The runner-up for the Team Collaboration Award was the “Artificial Intelligence in Spirometry” team. Students Catherine Tseng, Roshni Kumar, Andrew Li, Yiqing Zhang, and Samarth Ghai worked with Thomas Lee from the Fisher Center for Business Analytics at Haas School of Business to improve classification of clinical quality in spirometry tests. Spirometry tests test lung function to identify and manage chronic lung disease, and the team’s goal was to automatically detect errors and remedy them. As a continuing project from the previous Fall 2022 semester, this team explored data from the University of Washington to construct models of the distribution of errors and patient demographics.
Congratulations to all teams for successfully completing a semester with Data Science Discovery!