Want to make artificial intelligence systems safe? Design robots to continually ask and learn what humans want, a UC Berkeley expert said during a recent lecture.
These robots would defer to humans, looking for and acting on people’s feedback, described Anca Dragan. To succeed in developing safe systems, we must better emulate how humans behave, consider how different people’s values intersect in AI and assess the impact these systems will have on those values.
“Every problem is an assistance problem,” Dragan, an associate professor in Berkeley’s Department of Electrical Engineering and Computer Sciences (EECS), said in the Oct. 6 lecture. “It all comes down to understanding humans better from a computational perspective.”
Public awareness and the capabilities of AI systems sky-rocketed recently, as these technologies permeated everything from art to research. This spurred fears about the spread of misinformation, job displacement and more. Now government, business and labor decision-makers are grappling with the safety of these systems and the roles they play in society.
The event was the third this fall in a series on artificial intelligence sponsored by Center for Information Technology Research in the Interest of Society and the Banatao Institute (CITRIS), the Berkeley AI Research Lab and the College of Computing, Data Science, and Society (CDSS). EECS is shared by CDSS and Berkeley’s College of Engineering.
Re-define the problem, unlock capabilities
Robots didn’t work well even 15 years ago, Dragan said. Scientists over time have optimized algorithms and models to improve AI systems’ abilities.
As tasks become more complex, experts’ struggle to tell the robots what they want them to do specifically enough to consistently result in the actions humans want. Instead of designing a robot around a specific reward, Dragan suggested designing it around an intended one.
“The problem is that there's a person who wants something internally, and the robot's job is really to do what this person wants internally,” said Dragan. “This way of formulating the decision problem for the robot is a big key to unlocking the capability that we're largely missing of aligning AI agents to do what we want.”
This approach has several benefits, she said. It means the robot is designed to maintain uncertainty and act conservatively and to constantly seek human feedback and learn from it.
The design method is also flawed. For example, the premise hinges on the assumption that if the robot learns from the human feedback, its actions will reflect what humans want.
But that’s not true, Dragan said. Humans “don’t make choices in proportion to the cumulative reward,” she explained. According to a recent study, even a small flaw in this kind of human model can result in “catastrophic errors” about what the AI system learns and acts on, she said.
Take X, formerly known as Twitter. A generous interpretation is to think of its recommendation algorithm as trying to optimize for user happiness, looking at what people engage with as evidence of what brings them value, she said. In a study, users reviewed content they subscribe to versus content surfaced by the algorithm. The algorithm recommended tweets that would prompt emotional or angry responses, which was true for political content.
“It’s not actually helpful. It increases partisanship and, in general, it improves people's perception of their political in-group, and makes the perception of their political out-group worse,” said Dragan of the content. Users will “tell you that essentially, for all tweets, the algorithm is doing a good job bringing in… value. For political tweets? Not at all.”
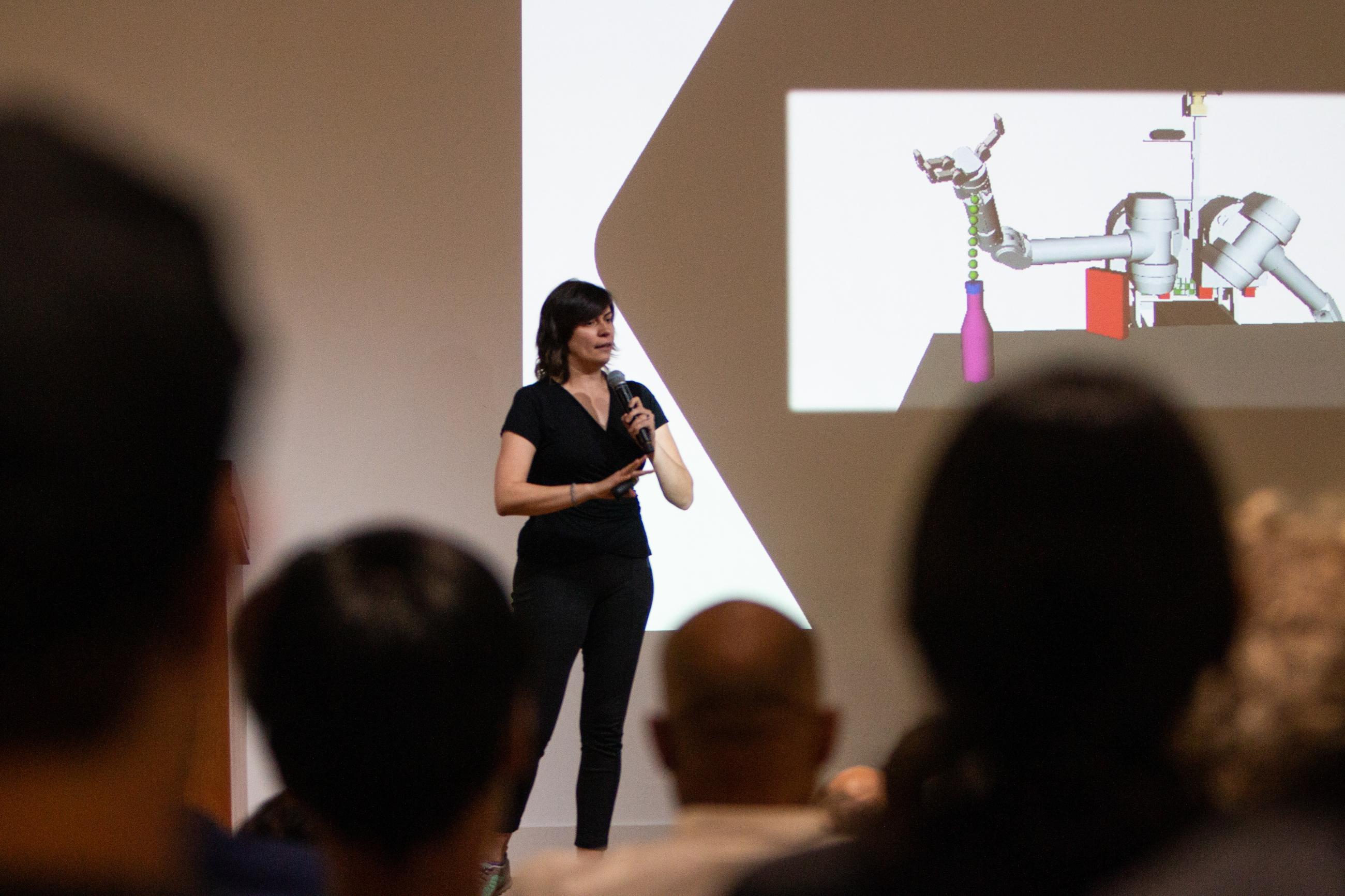
A bottleneck around human understanding
These kinds of flaws can be addressed, Dragan said. Scientists can create models that actually characterize how humans behave or at least get closer to that. For example, large language models could consider “false beliefs” – that someone may have beliefs that differ from the mainstream or from the belief that the AI agent holds about what is factual. This difference in what they believe might explain why they are choosing to act a certain way.
“I would sure love for a robot or an AI agent looking at a parent’s decision to not vaccinate their child for measles would not simply conclude that the parent fails to value their child’s health,” said Dragan.
The bottleneck to developing capable AI is different from 15 years ago, Dragan said. Before, scientists may not have had models of the physical world or known how to optimize these systems. Now “the bottleneck is more and more human understanding.”
"The bottleneck is more and more human understanding."
“It’s been surprising to me just how nuanced of an understanding of us agents need to have – not in order to be purely, raw capable – but in order to align that capability with what is actually useful and beneficial to us,” said Dragan.
To tackle these sorts of issues moving forward requires an adaptation of educational institutions to offer students more interdisciplinary opportunities, said Dragan. She has studied psychology as part of her AI research efforts and is now learning about citizen’s assemblies and the philosophy of choosing for changing selves.
“CDSS at Berkeley is trying to do a good job at crossing these AI plus X boundaries,” said Dragan. “I do think that collaborations are useful. But in my personal experience, I found that it really does take one person to understand enough about the other topic and internalize everything enough to be able to put it into their domain.”